Workflow
Workflow#
How does AI-Learn work? To develop a skills-based curriculum, an educator begins with the AI-Author workbench and design wizard.
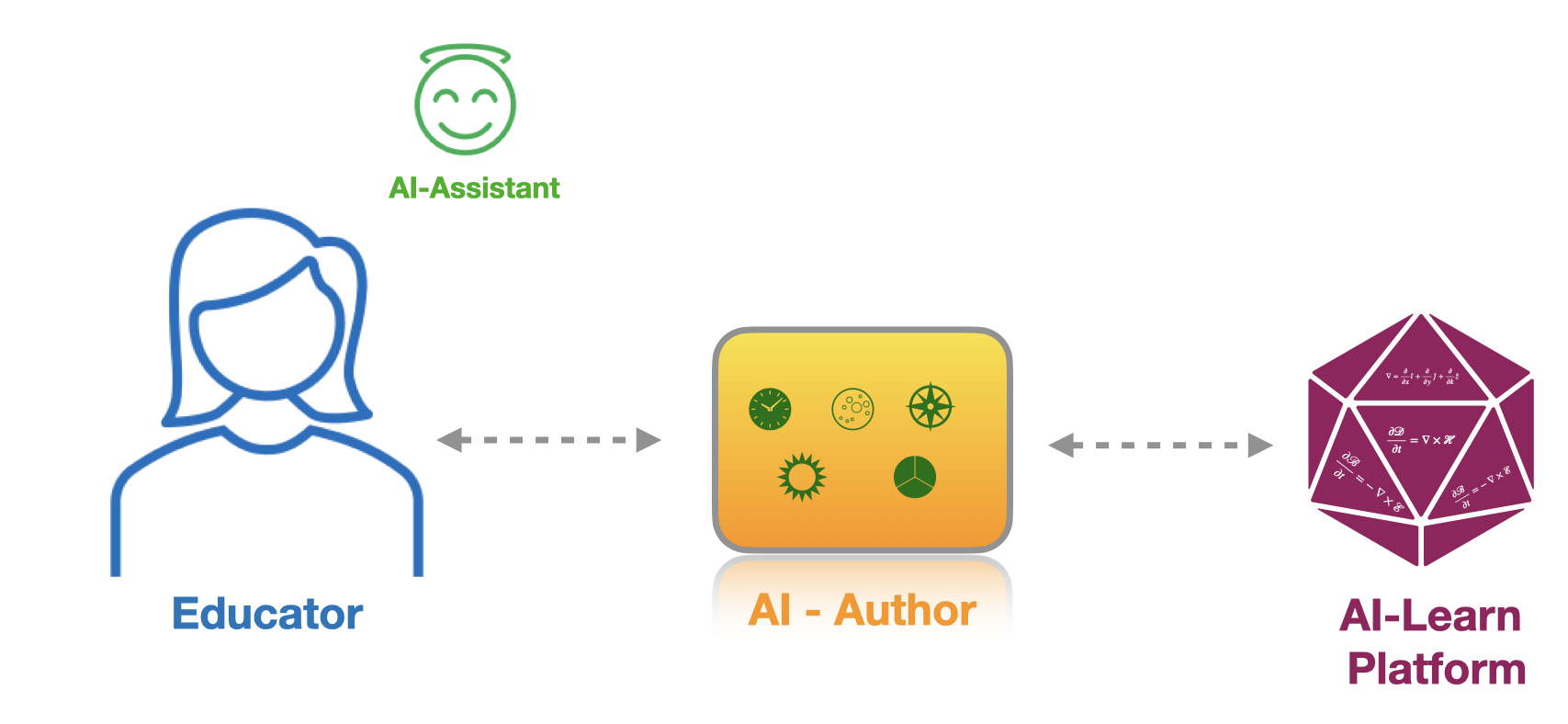
Fig. 3 How Does AI-Learn Work?#
The AI-Learn workflow consists of the following primary modes:
In extract mode, an educator automatically extracts a curriculum by issuing a series of guided prompts. AI-Learn then automatically generates the curriculum in the form of ALA. The work of extraction is performed behind the scenes by AI-Ada, an AI engine based on Large Language Models (LLMs). The extracted curriculum is populated in the design canvas for review and edit. AI-Learn can automatically extract 50-75% of the curriculum for most STEM fields without prior training. AI-Learn can also extract ALAs from proprietary digital materials or Open Educational Resources (OER) with additional modest training.
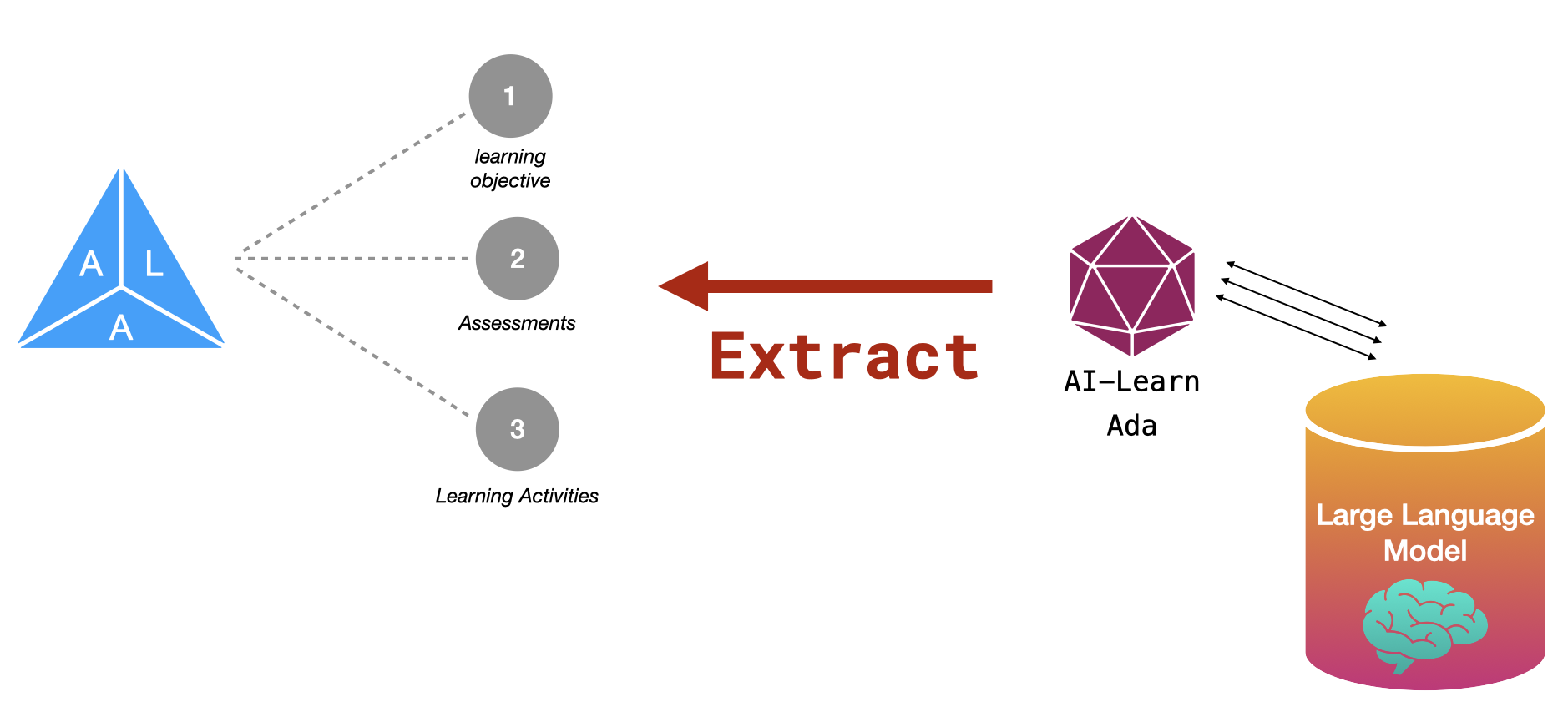
Fig. 4 ALA Automated Extraction from Large Language Model#
In edit mode, an educator uses AI-Author to create a modular curriculum from scratch or, more likely, edit a curriculum following extraction and discovery.
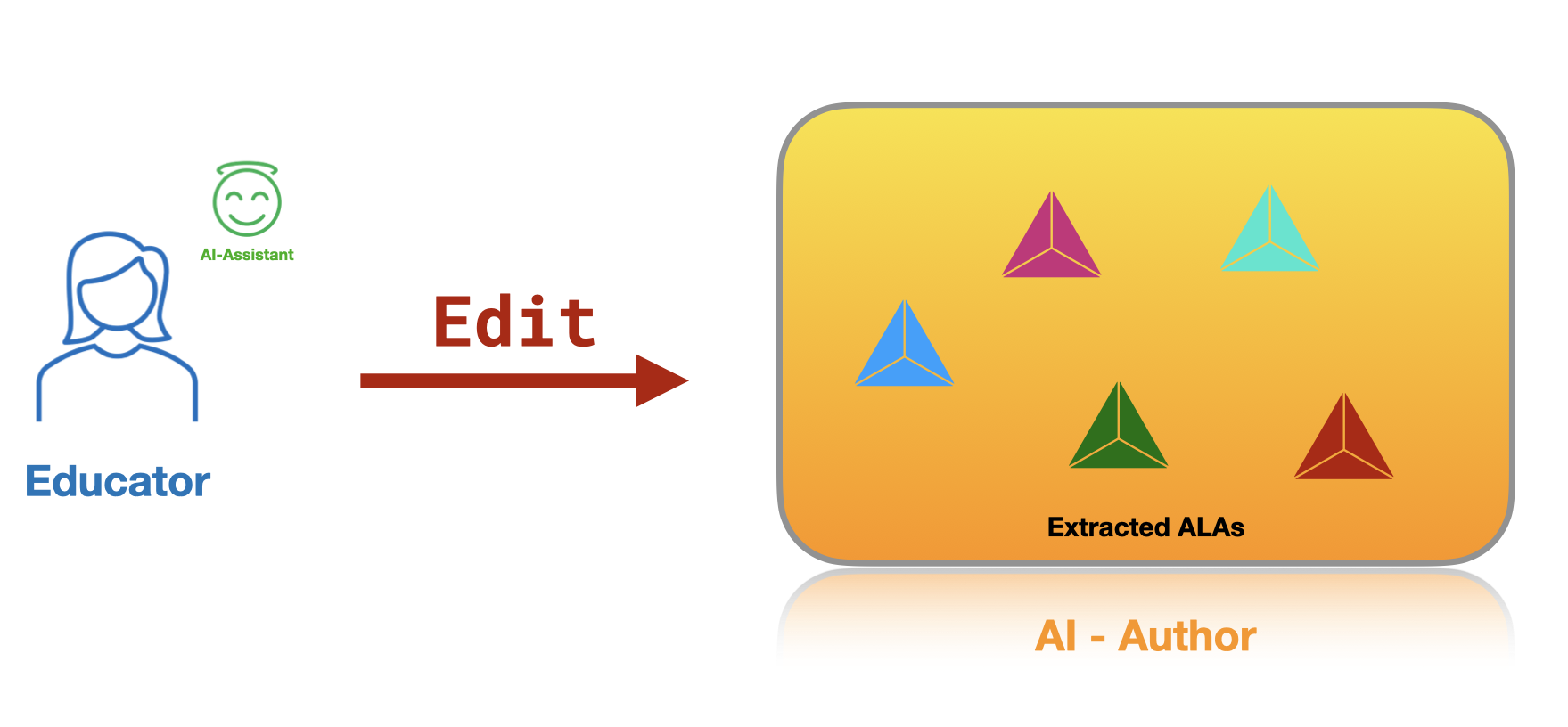
Fig. 5 Editing of ALAs in AI-Author#
In discover mode, an educator performs advanced search and discovery against a digital repository AI-Jorge. AI-Jorge contains pre-configured ALAs contributed by the educational community and curated by domain experts. The discovered ALAs are populated in the design canvas for review and edit.
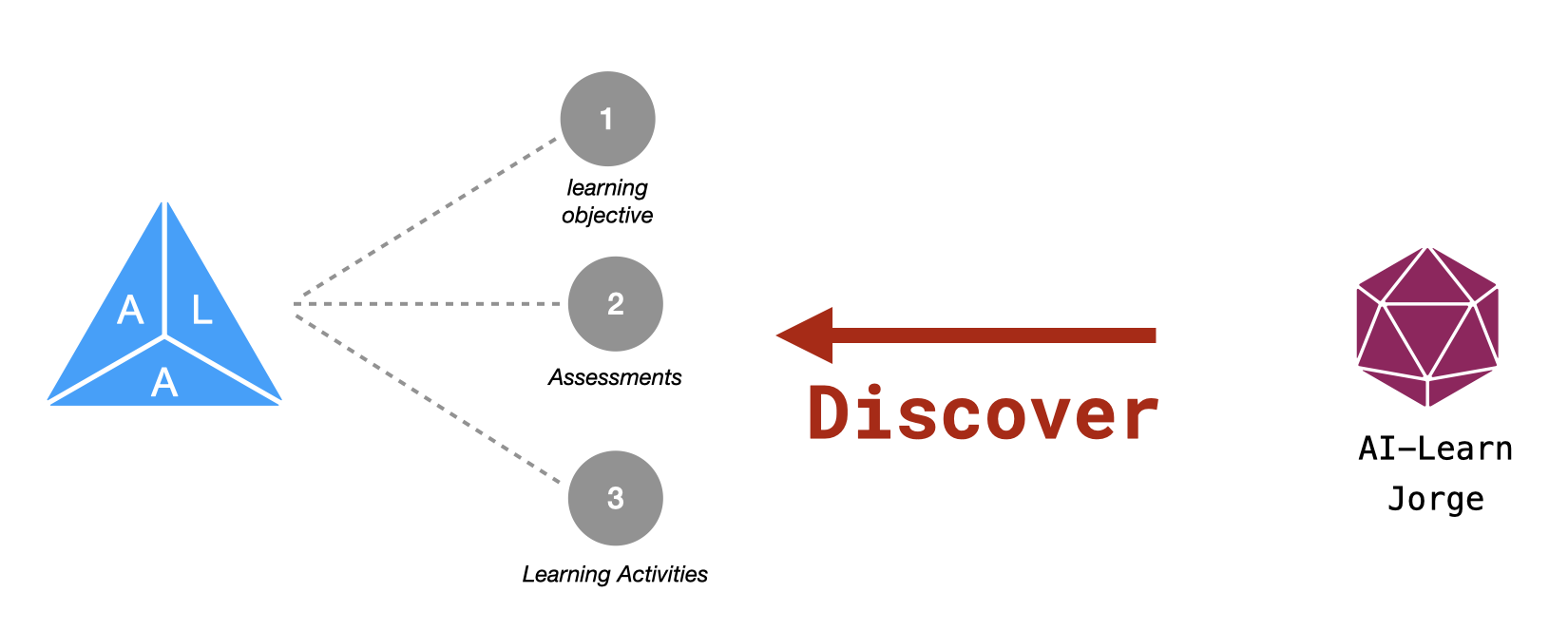
Fig. 6 Discovery of pre-configured ALAs in AI-Jorge#
In deploy mode, an educator deploys ALAs to intelligent tutoring systems and learning management systems using standard protocols such as 1EDTECH’s Learning Tools Interoperability (LTI). ALAs can also be deployed to AI-Emmy, a next-generation intelligent tutoring system.
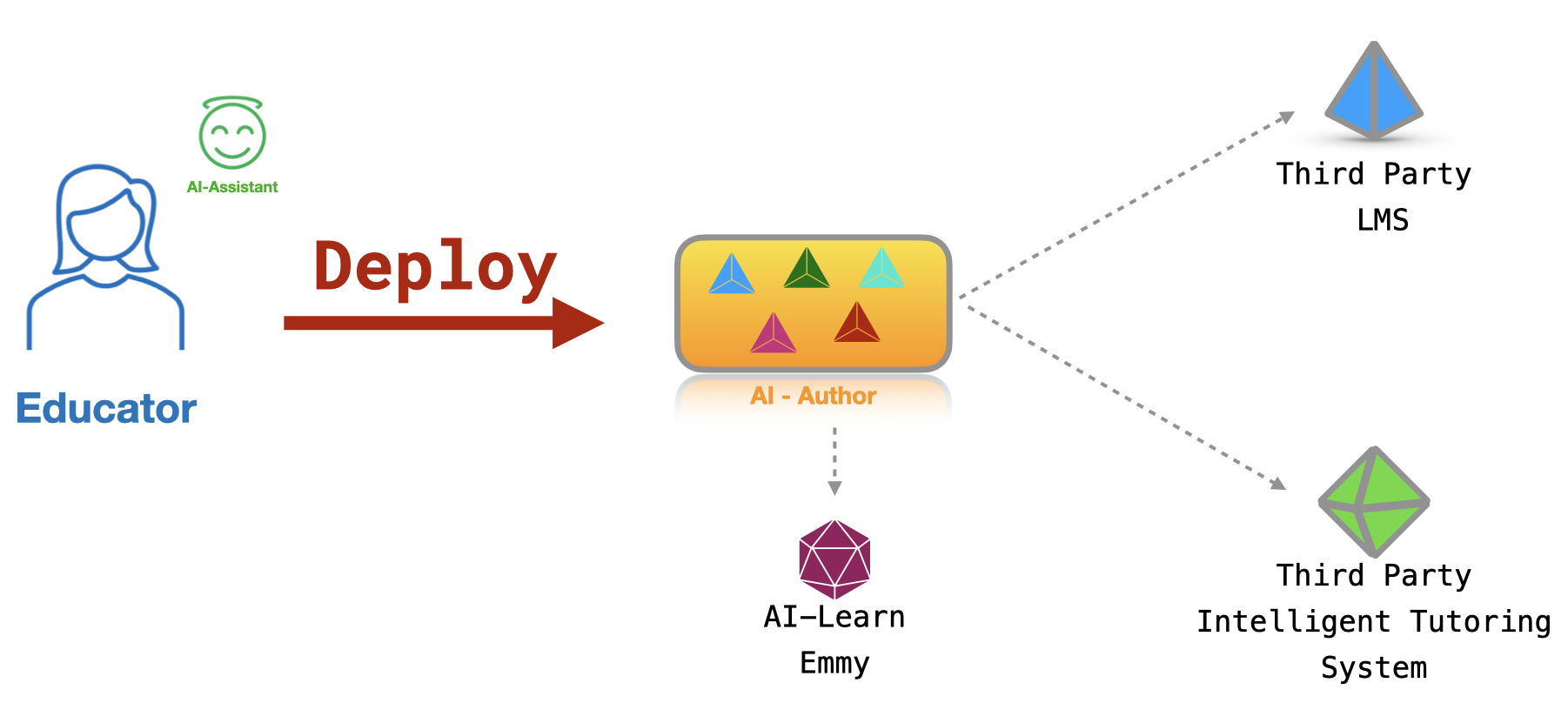
Fig. 7 Deployment of ALAs to Third party LMS, Intelligent Tutoring Systems and AI-Emmy#
In evaluate and improve mode, an educator evaluates ALAs using the AI-Learn analytics engine AI-Alonzo. The analytics ranges from simple methods such as item analysis to propensity modeling and causal inferencing. AI-Alonzo can also generates recommendation for improving ALAs based on observational data. ALA “improvements” are recorded in a “scientific logbook” of modifications under the structure of hypothesis-experiment-data.
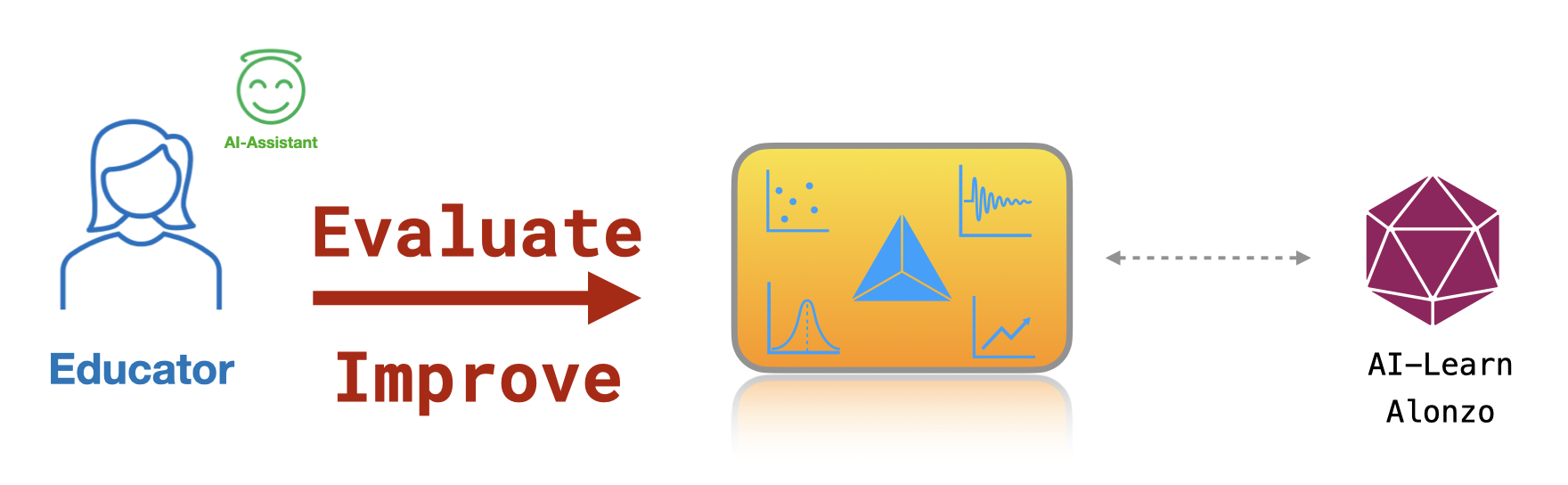
Fig. 8 Evaluation and Improvement of ALAs#
In share mode, an educator can share and license ALAs. AI-Jorge, therefore, is also a marketplace for exchanging ALAs based on license terms set by educators and institutions. Educators can also tag, rate, annotate, and recommend ALAs.
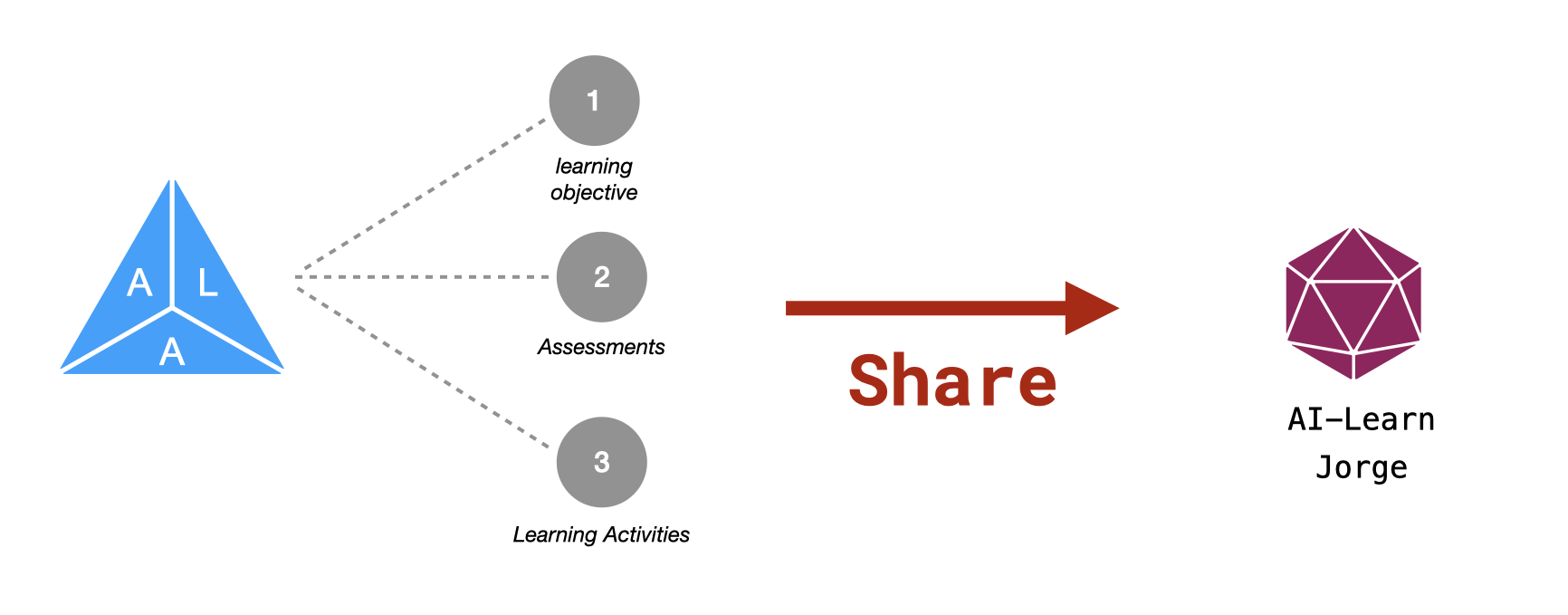
Fig. 9 Sharing of ALAs#